With 65% of businesses worldwide unable to analyze all the data they collect, the extensiveness of missed opportunities for innovation and performance is truly shocking. On the other end of the spectrum, 99% of Fortune 1000 companies reported investing heavily in data and AI initiatives in 2021. But even those firms that are still struggling with big data adoption can inspire other businesses with successful data analytics use cases resulting in:
- increased profit;
- cost reductions;
- higher operating margins;
- more effective strategic decisions;
- improved control of operational processes;
- a better understanding of customers;
- increased workforce productivity;
- enhanced security.
Yet, some executives don’t quite understand how data analytics apply to their business sectors, which has encouraged us to look into real-life big data analytics examples across industries.
Data analytics examples for OTT
- Personalized movie recommendation packages. While content recommendations save viewers’ time and, thus, improve the customer experience, personalization allows OTT media services to maximize the effect and increase user satisfaction even further. Advanced data analytics puts together users’ ratings, reviews, watch history, search queries, time spent watching a video, and other data to construct viewer profiles, embed them into content filtering, and provide customers with movies they are sure to like.
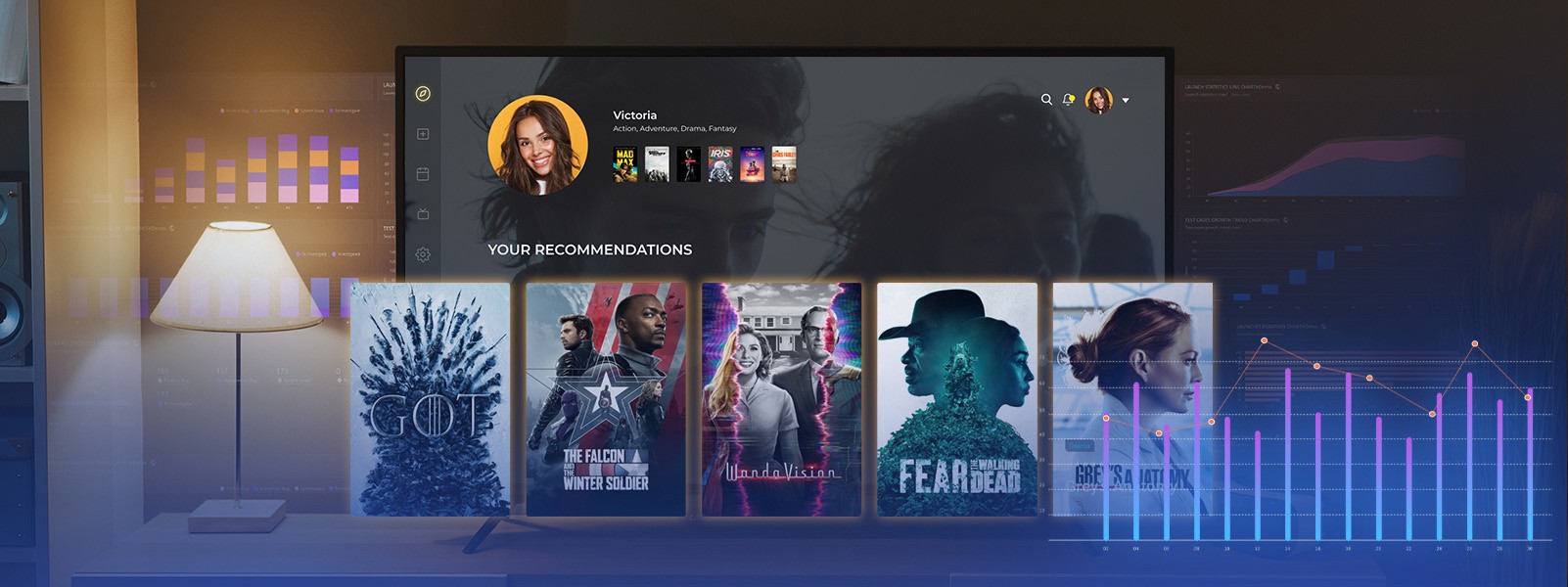
- Segmented marketing campaigns. With over 51% of all OTT market revenue coming from AVOD, attracting advertisers with precise ad delivery becomes crucial for content monetization in this highly competitive environment. Data analytics factors in viewers’ location, demographics, online behaviors, lifestyle, and interests to group the users into categories and show them the most relevant ads, which improves the click-through rates with reduced irritation on the customer side.
- Subscription package optimization. Guessing is not the best approach to pricing for either subscription-based or pay-per-view OTT services, and that’s just what they do when disregarding things like customer behavior, demographics, geographical market specifics, historical sales data, competition analysis, and demand fluctuations. All these pieces of data can be gathered and sifted to arrive at optimal pricing tiers and subscription packages tailored to target customers and their willingness to pay.
Case in point: Online video monitoring solution for an OTT TV platform
Is it possible to couple a solution to technical issues like infrastructure monitoring and video quality control with marketing efforts aimed at enhanced content acquisition and better monetization? The answer we gave to the owner of a US-based OTT TV platform was a decided “yes”.
Oxagile incorporated big data into scalable architecture capable of handling an unlimited number of users and presented a monitoring solution designed to process both historical and real-time data. Within 3 months after the launch, the client saw a 47% cost reduction and a 58% CTR boost thanks to well-rounded user behavior and distribution analysis.
AdTech data analytics use cases
- Media performance analysis. As adtech solutions are aimed at helping advertisers navigate today’s complex digital advertising landscape, they can’t fail to provide their users with data for measuring the effectiveness of campaigns delivered through different channels. Cross-channel data collection for attribution modeling is just one of the big data analytics use cases that allow advertisers to determine the value of different channels.
- Ad inventory management. No publisher will make great strides in content monetization without quantifying and pricing their assets correctly. Aggregating detailed analytics about audiences, preferences, prime time, demand trends, and other parameters translates into a clear understanding of ad slots available across a whole host of linear and digital channels and, as a result, better selling opportunities.
- Sentiment analysis and social listening. Advertisers want to protect their brands from negative associations, and adtech services that give them an effective tool for finding the right environments gain a competitive edge. Opinion mining and social listening work to extract and assess sentiments (positive, neutral, or negative), identify associated emotions (fear, joy, sadness, or hope), and analyze content with due regard to context for better brand suitability.
How we can help
Our team created an NLP-driven solution trained to define negative reviews, spam comments, and inappropriate content for a company offering brand management services to over 1,600 clients. We are ready to help your business leverage opinion mining and social listening and provide technology-powered care for brand reputation.
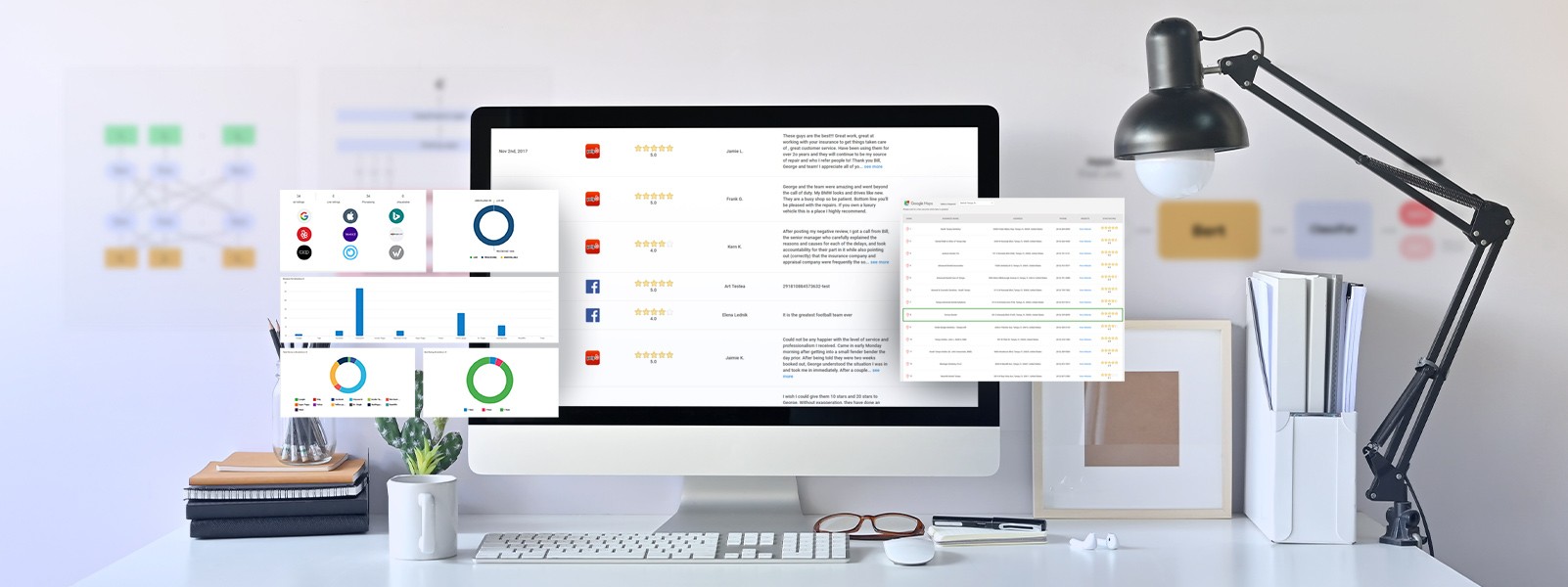
Data analytics use cases in EdTech
- Personalized assistance for students. Red-flagging students who are at risk of falling below acceptable performance levels is one of the data analytics use cases that help educational institutions generate a larger number of successful graduates and improve their reputation. Based on previous performance data and real-time progress tracking, algorithms built in LMSs can alert supervisors about possible failures so that they can step in with extra support and prevent poor academic performance and dropouts.
- Resource optimization. Since educational institutions have to manage not only students but also their academic staff, utilities, and other resources, edtech platforms can arm them with analytics solutions for better resource allocation. For example, trends derived from attendance statistics, teachers’ workload, and academic records for different classes are useful for optimizing curriculums and improving cost efficiency.
- Students’ engagement improvement. While maintaining students’ engagement was one of the greatest challenges during lockdowns, educators could, and still can, get a fix with valuable insights extracted from the depths of learning management systems. By combining engagement metrics with knowledge about students’ interests, they are able to create a learner-centric curriculum and introduce timely changes to the content to keep students committed and motivated.
Case in point: WebRTC-based proctoring platform
There is always some room for improvement, and sometimes it can make for a 93% rise in capacity. That was the outcome of Oxagile’s expertise applied to migrating a legacy proctoring solution to a modern tech stack complemented with workflow automation and advanced reporting.
The updated platform allowed the leading proctoring company providing its services in 129 countries to implement cutting-edge biometrics-assisted exam monitoring, facilitate communication between proctors and students through text and audio chats, and transform captured pieces of data into scoring functionality, performance metrics, real-time incidents reporting, and other actionable insights.
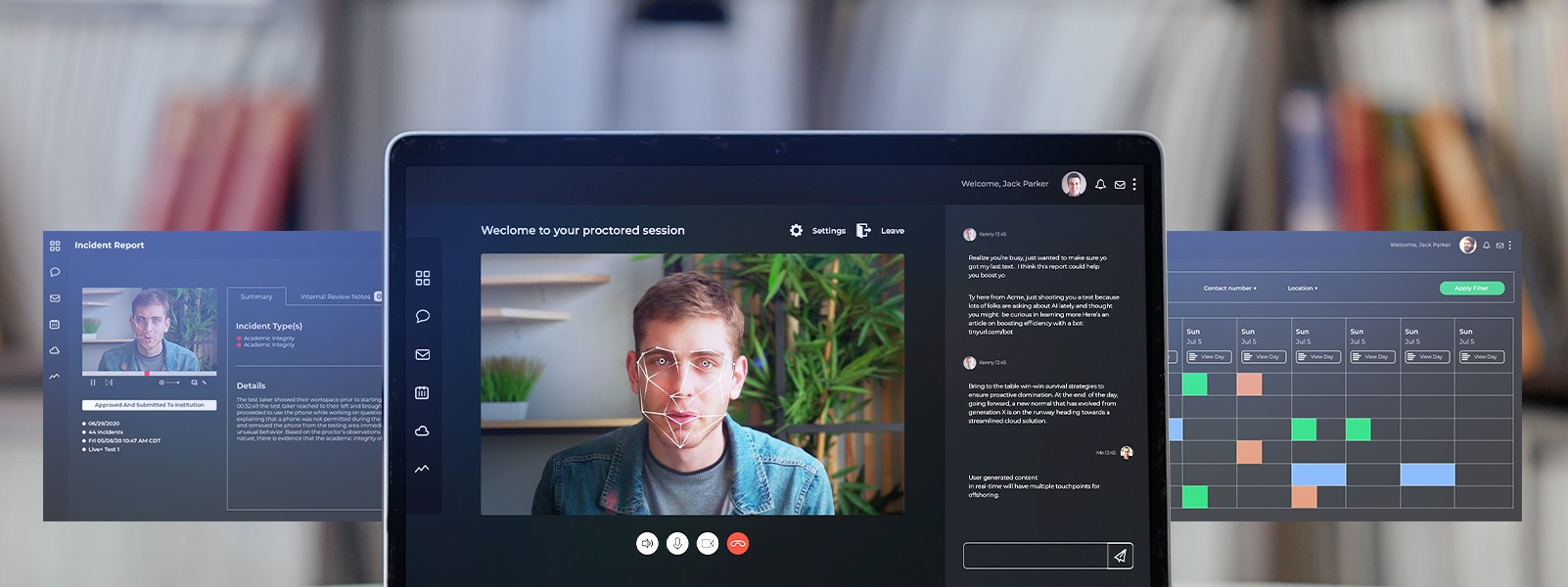
Ecommerce data analytics
- Intelligent SKU-level analysis. SKU analysis is not only about effective e-commerce catalog management and tracking inventory across multiple channels. With the help of data analytics tools, tracking inventory across multiple channels becomes more efficient, enabling businesses to optimize product availability and streamline stock management. Also, with the help of data analytics that combines historical sales performance with real-time data, online retailers can explore demand for products and play up fast-moving items to increase profitability or cut costs on inventory that doesn’t get sold by avoiding excessive SKU proliferation.
- Customer lifetime value predictions. Some e-commerce patrons are more valuable than others because they are likely to spend more money over the longest period. Obviously, they require particular care from sales teams and customer service, but marketers have to identify them first. By anatomizing customer behavior, purchase history, spending patterns, and transaction data, analytics tools provide online retailers with accurate CLV predictions to miss no one from those best buyers.
- Customer churn prevention. Since customer acquisition incurs higher costs than customer retention, data analytics examples aimed at churn prevention deserve special attention. Modern analytics tools can uncover signs of dissatisfaction within the clientage of e-commerce platforms and even dig into customer segmentation to discover whole at-risk groups. They aggregate data from social media, website visits, call logs, and other company interactions to let decision-makers proactively handle the issue.
Case in point: An intelligent product recommendation system for e-commerce
Catering to the needs of e-shoppers for better customer retention and increased sales doesn’t need to be tricky for e-commerce content managers. Wouldn’t you want to match customer preferences with products from your catalog using a simple drag-and-drop feature?
Oxagile has already made this dream come true with an intelligent product recommendation system featuring a powerful statistics module that provides valuable insights into shopper choices.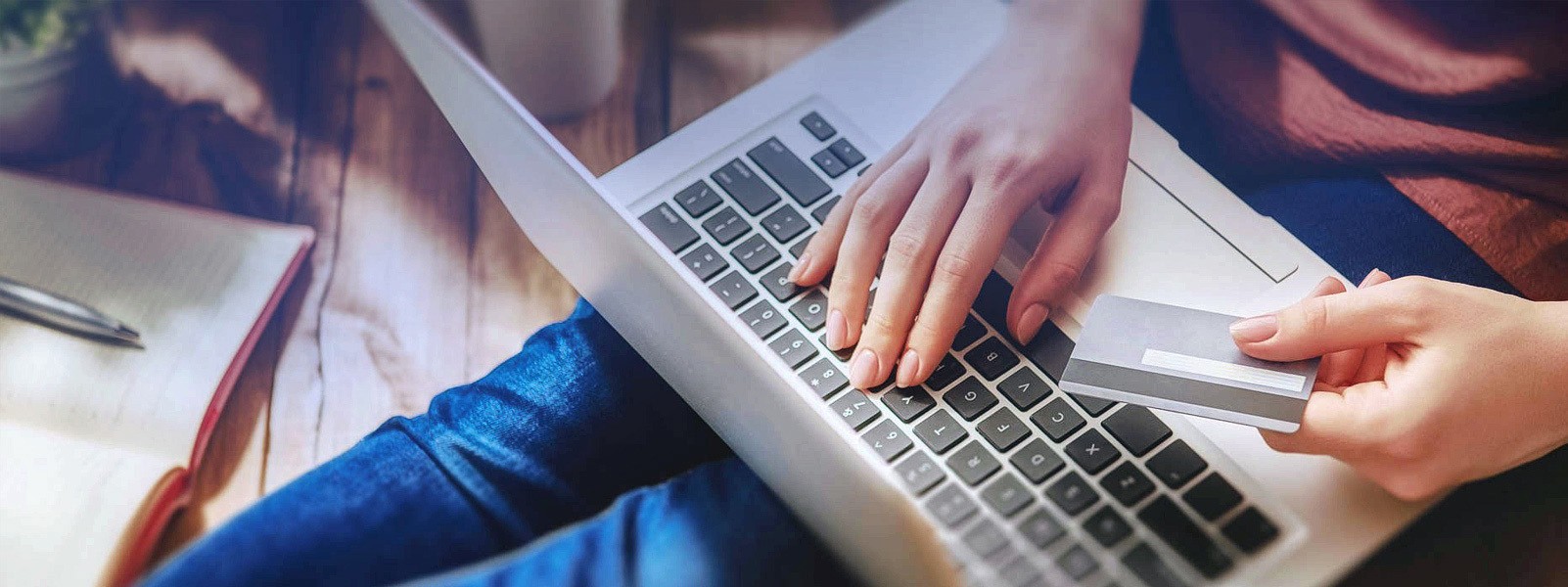
Big data solutions for Retail
- Market basket analysis. There is hardly a retailer that doesn’t want to increase their revenue by cross-selling and up-selling. Data analytics use cases cover these areas perfectly by applying predictive analytics to sift through purchase history and provide suggestions on products that might be combined to appeal to customers. Retailers can also drive higher sales by developing new product offerings with differential market basket analysis, which focuses on revealing exceptions in buying patterns. For companies looking to streamline stock levels and reduce costs, inventory management solutions offer valuable insights, enabling efficient tracking and optimization of product availability.
- Foot traffic data. While measuring in-store foot traffic is helpful for a better understanding of the customer base and product mix efficacy, retailers will also benefit from analyzing external foot traffic when trying to optimize their current store locations or find the best place for a new shop. The required data can be sourced from demographics and data aggregators and transformed into actionable insights for locating target markets and revealing better investment opportunities.
- New product launches. Although no product launch goes as smoothly as we’d like it to, data-driven product development is among the truly game-changing data analytics use cases. Retailers can build predictive models for new products and services by defining attributes of past and current products and linking these attributes to the commercial success of the offerings. Further modeling through focus groups and test markets will help them anticipate customer demand, streamline a product launch, and optimize initial sales.
Big data in Finance
- Insider threat and fraud detection. The financial sector has always been a primary target for fraud and data theft, with the number of incidents growing by 44% over the last two years alone. This is yet another area to demonstrate big data analytics use cases, specifically, for recognizing fraudulent behavior, intercepting spam or suspicious calls, text messages, and emails, detecting abnormalities across users and endpoints, generating risk scores, and delivering real-time alerts to prevent or at least mitigate possible damage.
- Financial risk modeling. While the COVID-19 crisis shook the capital, profit-and-loss, and liquidity positions of many financial institutions, they are now on the brink of the next economic slowdown, which calls for using advanced data analytics in risk modeling. It can spot potential areas of risk in the massive data silos accumulated by financial organizations and identify trends suggesting the development of worst-case scenarios that can affect businesses and their bottom lines.
- Next best action recommendations. Apart from strong security, financial services and banking organizations must deliver the best customer experience to improve sales, which can be done by implementing a client-centric approach. As such, it will be smart to equip their reps with next-best-action recommendations powered by predictive analytics and based on both the customer’s needs and the financial institution’s policies. The recommendations will suggest the most effective action, like making an offer or reaching out by phone, to achieve the desired outcomes and optimize the customer journey.
Healthcare data analytics
- Healthcare infrastructure improvement. Managing demand for hospital beds and other healthcare resources in an effective way is obviously a less-expensive alternative to building new capacity. However, the task is highly complicated since it involves plenty of variables, such as the existing number of operating rooms and beds, treatment and nursing plans, patient age, gender, or condition, and more. Data insights obtained with the help of analytic tools will make planning and management much easier and more accurate.
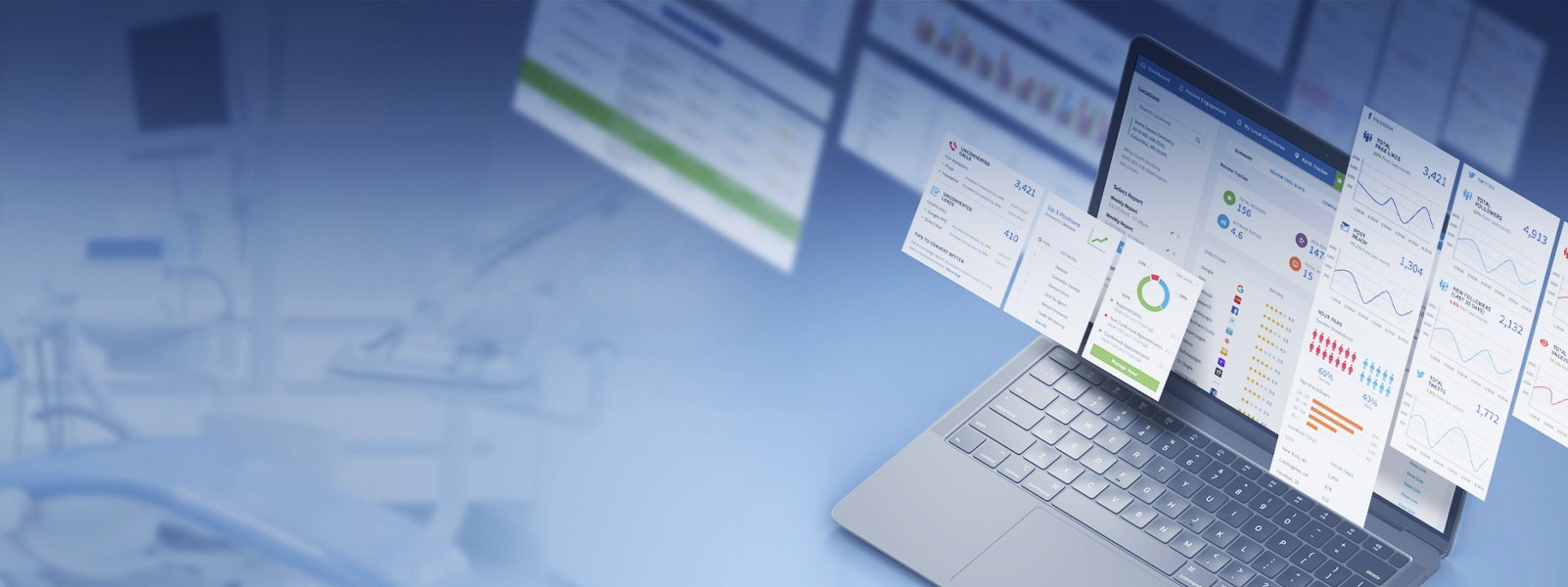
- Personalized treatment. Personalized care based on clinical data pulled from diverse sources and used to create a panoramic view of a patient’s state of health brings many benefits, starting from value added to medical services and ending with lower readmission rates. Data analyzed in real-time, like alerts triggered by an increase in blood sugar levels, unlock even greater potential of analytics tools incorporated into medical treatment.
- Billing and claim analytics. Data analytics use cases for the healthcare industry go far beyond clinical and operational areas, helping caregivers discover lost revenue opportunities in billing and claims data. Namely, while claim denials cost hospitals billions of dollars, data analytics has the power to predict a denial and cut costs on reclaims by identifying missing information, validating codes, revealing duplicate claims, verifying coverage, and checking the accuracy of fee schedules.
Summing up
Although it’s tricky to fit all possible data analytics examples into one article, the diversity and value of the considered use cases should convince business leaders in different fields to rethink their current data utilization and incorporate cutting-edge analytics tools into their business processes. And if you need expert assistance in overcoming big data challenges, don’t hesitate to get in touch with our specialists.